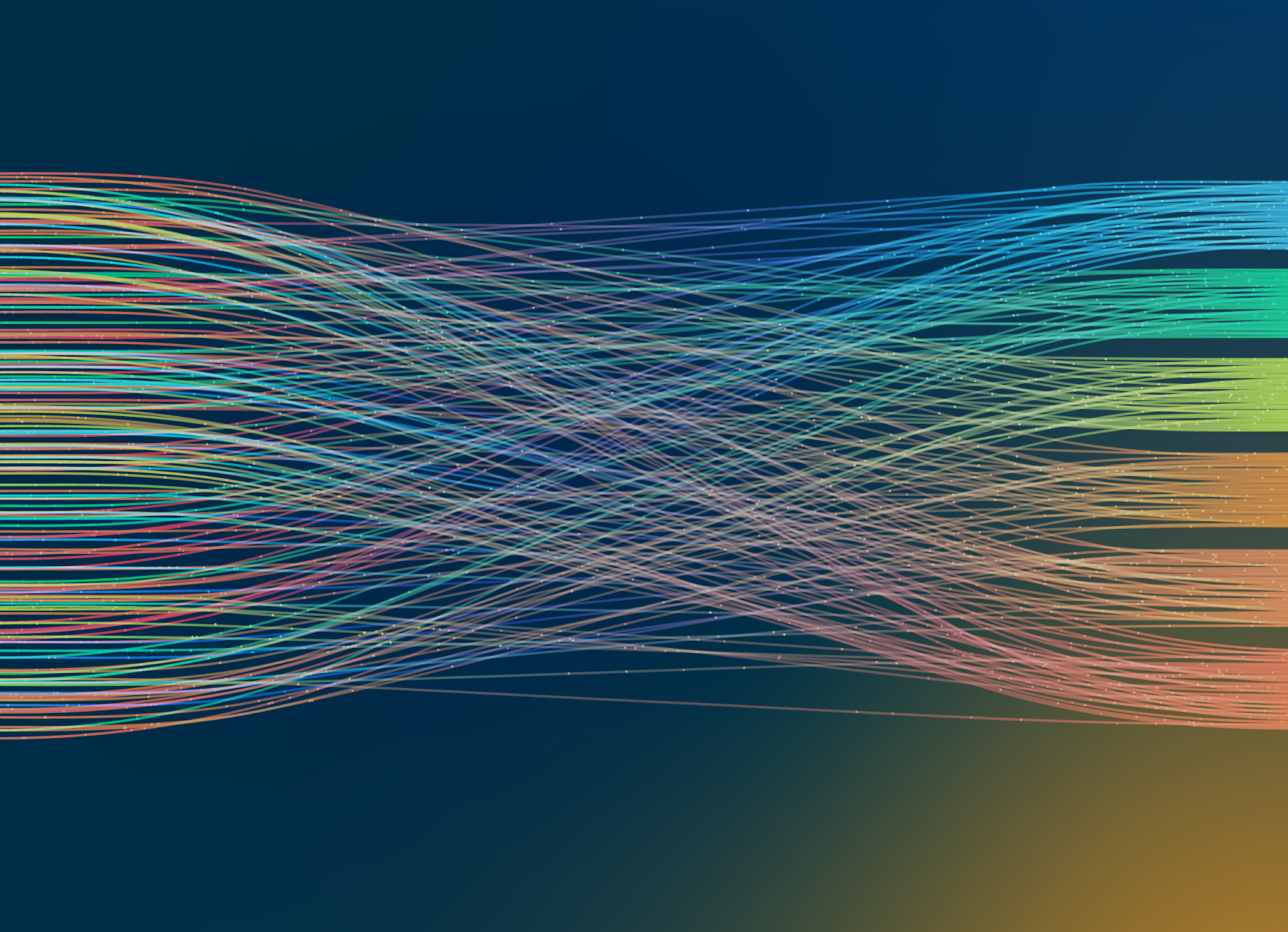
Executive Summary
International cooperation on artificial intelligence—why, what, and how
Since 2017, when Canada became the first country to adopt a national AI strategy, at least 60 countries have adopted some form of policy for artificial intelligence (AI). The prospect of an estimated boost of 16 percent, or US$13 trillion, to global output by 2030 has led to an unprecedented race to promote AI uptake across industry, consumer markets, and government services. Global corporate investment in AI has reportedly reached US$60 billion in 2020 and is projected to more than double by 2025.
At the same time, the work on developing global standards for AI has led to significant developments in various international bodies. These encompass both technical aspects of AI (in standards development organizations (SDOs) such as the International Organization for Standardization (ISO), the International Electrotechnical Commission (IEC), and the Institute of Electrical and Electronics Engineers (IEEE) among others) and the ethical and policy dimensions of responsible AI. In addition, in 2018 the G-7 agreed to establish the Global Partnership on AI, a multistakeholder initiative working on projects to explore regulatory issues and opportunities for AI development. The Organization for Economic Cooperation and Development (OECD) launched the AI Policy Observatory to support and inform AI policy development. Several other international organizations have become active in developing proposed frameworks for responsible AI development.
In addition, there has been a proliferation of declarations and frameworks from public and private organizations aimed at guiding the development of responsible AI. While many of these focus on general principles, the past two years have seen efforts to put principles into operation through fully-fledged policy frameworks. Canada’s directive on the use of AI in government, Singapore’s Model AI Governance Framework, Japan’s Social Principles of Human-Centric AI, and the U.K. guidance on understanding AI ethics and safety have been frontrunners in this sense; they were followed by the U.S. guidance to federal agencies on regulation of AI and an executive order on how these agencies should use AI. Most recently, the EU proposal for adoption of regulation on AI has marked the first attempt to introduce a comprehensive legislative scheme governing AI.
Global corporate investment in AI has reportedly reached US$60 billion in 2020 and is projected to more than double by 2025.
In exploring how to align these various policymaking efforts, we focus on the most compelling reasons for stepping up international cooperation (the “why”); the issues and policy domains that appear most ready for enhanced collaboration (the “what”); and the instruments and forums that could be leveraged to achieve meaningful results in advancing international AI standards, regulatory cooperation, and joint R&D projects to tackle global challenges (the “how”). At the end of this report, we list the topics that we propose to explore in our forthcoming group discussions.
Why international cooperation on AI is important
Even more than many domains of science and engineering in the 21st century, the international AI landscape is deeply collaborative, especially when it comes to research, innovation, and standardization. There are several reasons to sustain and enhance international cooperation.
- AI research and development is an increasingly complex and resource-intensive endeavor, in which scale is an important advantage. Cooperation among governments and AI researchers and developers across national boundaries can maximize the advantage of scale and exploit comparative advantages for mutual benefit. An absence of international cooperation would lead to competitive and duplicative investments in AI capacity, creating unnecessary costs and leaving each government worse off in AI outcomes. Several essential inputs used in the development of AI, including access to high-quality data (especially for supervised machine learning) and large-scale computing capacity, knowledge, and talent, benefit from scale.
- International cooperation based on commonly agreed democratic principles for responsible AI can help focus on responsible AI development and build trust. While much progress has been made aligning on responsible AI, there remain differences—even among Forum for Cooperation on AI (FCAI) participants. The next steps in AI governance involve translating AI principles into policy, regulatory frameworks, and standards. These will require deeper understanding of how AI works in practice and working through the operation of principles in specific contexts and in the face of inevitable tradeoffs, such as may arise when seeking AI that is both accurate and explainable. Effective cooperation will require concrete steps in specific areas, which the recommendations of this report aim to suggest.
- When it comes to regulation, divergent approaches can create barriers to innovation and diffusion. Governments’ efforts to boost domestic AI development around concepts of digital sovereignty can have negative spillovers, such as restrictions on access to data, data localization, discriminatory investment, and other requirements. Likewise, diverging risk classification regimes and regulatory requirements can increase costs for businesses seeking to serve the global AI market. Varying governmental AI regulations may necessitate building variations of AI models that can increase the work necessary to build an AI system, leading to higher compliance costs that disproportionately affect smaller firms. Differing regulations may also force variation in how data sets are collected and stored, creating additional complexity in data systems and reducing the general downstream usefulness of the data for AI. Such additional costs may apply to AI as a service as well as hardware-software systems that embed AI solutions, such as autonomous vehicles, robots, or digital medical devices. Enhanced cooperation is key to create a larger market in which different countries can try to leverage their own competitive advantage. For example, the EU seeks to achieve a competitive advantage in “industrial AI”: EU enterprises could exploit that AI without the prospect of having to engage in substantial reengineering to meet requirements of another jurisdiction.
- Aligning key aspects of AI regulation can enable specialized firms in AI development to thrive. Such companies generate business by developing expertise in a specialized AI system, then licensing these to other companies as one part of a broader tool. As AI becomes more ubiquitous, complex stacks of specialized AI systems may emerge in many sectors. A more open global market would allow a company to take advantage of digital supply chains, using a single product with a natural language model built in Canada, a video analysis algorithm trained in Japan, and network analysis developed in France. Enabling global competition by such specialized firms will encourage healthier markets and more AI innovation.
- Enhanced cooperation in trade is essential to avoid unjustified restrictions to the flow of goods and data, which would substantially reduce the prospective benefits of AI diffusion. While the strategic importance of data and sovereignty has in many countries given rise to legitimate industrial policy initiatives aimed at mapping and reducing dependencies on the rest of the world, protectionist measures can jeopardize global cooperation, impinge on global value chains, and negatively affect consumer choice, thereby reducing market size and overall incentives to invest in meaningful AI solutions.
- Enhanced cooperation is needed to tap the potential of AI solutions to address global challenges. No country can “go it alone” in AI, especially when it comes to sharing data and applying AI to tackle global challenges like climate change or pandemic preparedness. The governments involved in the FCAI share interests in deploying AI for global social, humanitarian, and environmental benefit. For example, the EU is proposing to employ AI to support its Green Deal, and the G-7 and GPAI have called for harnessing AI for U.N. Sustainable Development Goals. Collaborative “moonshots” can pool resources to leverage the potential of AI and related technologies to address key global problems in domains such as health care, climate science, or agriculture at the same time as they provide a way to test approaches to responsible AI together.
- Cooperation among likeminded countries is important to reaffirm key principles of openness and protection of democracy, freedom of expression, and other human rights. The risks associated with the unconstrained use of AI solutions by techno-authoritarian regimes— such as China’s—expose citizens to potential violations of human rights and threaten to split cyberspace into incompatible technology stacks and fragment the global AI R&D process.
The fact that international cooperation is an element of most governments’ AI strategies indicates that governments appreciate the connection between AI development and collaboration across borders. This report is about concrete ways to realize this connection.
Related Content
At the same time, international cooperation should not be interpreted as complete global harmonization: countries legitimately differ in national strategic priorities, legal traditions, economic structures, demography, and geography. International collaboration can nonetheless create the level playing field that would enable countries to engage in fruitful “co-opetition” in AI: agreeing on basic principles and when possible seeking joint outcomes, but also competing for the best solutions to be scaled up at the global level. Robust cooperation based on common principles and values is a foundation for successful national development of AI.
Rules, standards, and R&D projects: Key areas for collaboration
Our exploration of international AI governance through roundtables, other discussions, and research led us to identify three main areas where enhanced collaboration would provide fruitful: regulatory policies, standard-setting, and joint research and development (R&D) projects. Below, we summarize ways in which cooperation may unfold in each of these areas, as well as the extent of collaboration conceivable in the short term as well as in the longer term.
Cooperation on regulatory policy
AI policy development is in the relatively early stages in all countries, and so timely and focused international cooperation can help align AI policies and regulations.
International regulatory cooperation has the potential to reduce regulatory burdens and barriers to trade, incentivize AI development and use, and increase market competition at the global level. That said, countries differ in legal tradition, economic structure, comparative advantage in AI, weighing of civil and fundamental rights, and balance between ex ante regulation and ex post enforcement and litigation systems. Such differences will make it difficult to achieve complete regulatory convergence. Indeed, national AI strategies and policies reflect differences in countries’ willingness to move towards a comprehensive regulatory framework for AI. Despite these differences, AI policy development is in the relatively early stages in all countries, and so timely and focused international cooperation can help align AI policies and regulations.
Against this backdrop, it is reasonable to assume that AI policy development is less embedded in pre-existing legal tradition or frameworks at this stage, and thus that international cooperation in this field can achieve higher levels of integration. The following areas for cooperation emerged from the FCAI dialogues and our other explorations.
- Building international cooperation into AI policies. FCAI governments should give effect to their recognition of the need for international engagement on AI by committing to pursue coordination with each other and other international partners prior to adopting domestic AI initiatives.
- A common, technology-neutral definition of AI for regulatory purposes. Based on the definitions among FCAI participants and the work of the OECD expert group, converging on a common definition of AI and working together to gradually update the description of an AI system, and its possible configurations and techniques, appears feasible and already partly underway. A common definition is important to guide future cooperation in AI and determines the level of ambition that can be reached by such a process.
- Building on a risk-based approach to AI regulation. A variety of governments and other bodies have endorsed a risk-based approach to AI in national strategies and in bilateral or multilateral contexts. Most notably, a risk-based approach is central to the policy frameworks of the two most prominent exemplars of AI policy development—the U.S. and the EU. These recent, broadly parallel developments have opened the door to developing international cooperation on ways to address risks while maximizing benefits. However, there remain challenges to convergence on a risk-based approach. Dialogue on clear identification and classification of risks, approaches to benefit-risk analysis, possible convergence on cases in which the risks are too high to be mitigated, and the type of risk assessment to be performed and who should perform it, would greatly benefit cooperation on a risk-based approach.
- Sharing experiences and developing common criteria and standards for auditing AI systems. The field of accountability in AI and algorithms has been the subject of wide and valuable work by civil society organizations as well as governments. The exchange of good practices and—ultimately—a common, or at least a compatible, framework for AI auditing would eliminate significant barriers to the development of a truly international market for AI solutions. It also would facilitate the emergence of third-party auditing standards and an international market for AI auditing, with potential benefits in terms of quality, price, and access for auditing services for deployers of AI. Additionally, exchange of practices and international standards for AI auditing, monitoring, and oversight would significantly help the policy community keep up to speed in market monitoring.
- A joint platform for regulatory sandboxes. Even without convergence on risk assessments or regulatory measures, an international platform for regulatory learning involving all governments that participate in FCAI and possibly others is a promising avenue for deepening international cooperation on AI. Such a platform could host an international repository of ongoing experiments on AI-enabled innovations, including regulatory sandboxes. As use of sandboxes becomes a more common way for governments to test the viability and conformity of new AI solutions under legislative and regulatory requirements, updating information on ongoing government initiatives could save resources and inform AI developers and policymakers. Aligning the criteria and overall design of AI sandboxes in different administrations could also increase the prospective benefits and impact of these processes, as developers willing to enter the global market might be able to go through the sandbox process in a single participating country.
- Cooperation on AI use in government: procurement and accountability. A natural candidate for further exchange and cooperation in FCAI is the adoption of AI solutions in government, including both “back office” solutions and more public-facing applications. The sharing of good practices and overall lessons on what works when deploying AI in government would also be an important achievement. Important areas in this respect are procurement and effective oversight of deployment.
- Sectoral cooperation on AI use cases. A sector-specific approach can ensure higher levels of regulatory certainty. In sectors like finance, key criteria such as fairness, discrimination, and transparency have long been subject to extensive regulatory intervention, and sectoral regulation must ensure continuity while accounting for the increasing use of AI. In health and pharmaceuticals, the use of AI both as a stand-alone solution and embedded in medical devices has prompted a very specific, technical discussion regarding the risk-based approach to be adopted and has already enabled valuable sectoral initiatives. The adoption of different standards and criteria in sectoral regulation may increase regulatory costs for developers willing to serve more than one sector and country with their AI solutions. In such a cross-cutting framework, examples from mature areas of regulation such as finance and health can also become a form of regulatory sandbox to model regulation for other sectors in the future.
Cooperation on sharing data across borders
Data governance is a focal area for international cooperation on AI because of the importance of data as an input for AI R&D and because of the added complexity of regulatory regimes already in place that restrict certain information flows, including data protection and intellectual property laws. Effective international cooperation on AI needs a robust and coherent framework for data protection and data sharing. There are a variety of channels addressing these issues including the Asia-Pacific Economic Cooperation group, the working group on data governance of the Global Partnership on AI, and bilateral discussions between the EU and U.S. Nonetheless, the potential impact of such laws on data available for AI-driven medical and scientific research requires specific focus as the EU both reviews its General Data Protection Regulation and considers new legislation on private and public sector data sharing.
There are other significant data governance issues that may benefit from pooled efforts across borders that, by and large, are the subject of international cooperation. Key areas in this respect include opening government data including international data sharing, improving data interoperability, and promoting technologies for trustworthy data sharing.
Cooperation on international standards for AI
As countries move from developing frameworks and policies to more concrete efforts to regulate AI, demand for AI standards will grow. These include standards for risk management, data governance, and technical documentation that can establish compliance with emerging legal requirements. International AI standards will also be needed to develop commonly accepted labeling practices that can facilitate business-to-business (B2B) contracting and to demonstrate conformity with AI regulations; address the ethics of AI systems (transparency, neutrality/lack of bias, etc.); and maximize the harmonization and interoperability for AI systems globally. International standards from standards development organizations like the ISO/IEC and IEEE can help ensure that global AI systems are ethically sound, robust, and trustworthy, that opportunities from AI are widely distributed, and that standards are technically sound and research-driven regardless of sector or application.
International standards from standards development organizations like the ISO/IEC and IEEE can help ensure that global AI systems are ethically sound, robust, and trustworthy, that opportunities from AI are widely distributed, and that standards are technically sound and research-driven regardless of sector or application.
The governments participating in the FCAI recognize and support industry-led standards setting. While there are differences in how the FCAI participants engage with industry-led standards bodies, a common element is support for the central role of the private sector in driving standards. That said, there is a range of steps that FCAI participants can take to strengthen international cooperation in AI standards. The approach of FCAI participants that emphasizes an industry-led approach to developing international AI standards contrasts with the overall approach of other countries, such as China, where the state is at the center of standards making activities. The more direct involvement by the Chinese government in setting standards, driving the standards agenda, and aligning these with broader Chinese government priorities requires attention by all FCAI participants with the aim of encouraging Chinese engagement in international AI standard-setting consistent with outcomes that are technically robust and industry driven.
Sound AI standards can also support international trade and investment in AI, expanding AI opportunity globally and increasing returns to investment in AI R&D. The World Trade Organization (WTO) Technical Barriers to Trade (TBT) Agreement’s relevance to AI standards is limited by its application only to goods, whereas many AI standards will apply to services. Recent trade agreements have started to address AI issues, including support for AI standards, but more is needed. An effective international AI standards development process is also needed to avoid bifurcated AI standards—centered around China on the one hand and the West on the other. Which outcome prevails will to some extent depend on progress in effective international AI standards development.
R&D cooperation: Selecting international AI projects
Productive discussion of AI ethics, regulation, risks, and benefits requires use cases because the issues are highly contextual. As a result, AI policy development has tended to move from broad principles to specific sectors or use cases. Considering this need, we suggest that developing international cooperation on AI would benefit from putting cooperation into operation with specific use cases. To this end, we propose that FCAI participants expand efforts to deploy AI on important global problems collectively by working toward agreement on joint research aimed at a specific development project (or projects). Such an effort could stimulate development of AI for social benefit and also provide a forcing function for overcoming differences in approaches to AI policy and regulation.
Criteria for the kinds of goals or projects to consider include the following:
- Global significance. The project should be aimed at important global issues that demand transnational solutions. The shared importance of the issues should give all participants a common stake and, if successful, could contribute toward global welfare.
- Global scale. The problem and the scope of the project should require resources on a large enough scale that the pooled support of leading governments and institutions adds significant value.
- A public good. Given its significance and scale, the project would amount to a public good. In turn, the output of the project should also be a public good and both the project and the output should be available to all participants and less developed countries.
- A collaborative test bed. Governance of the project is likely to necessitate addressing regulatory, ethical, and risk questions in a context that is concrete and in which the participants have incentives to achieve results. It would amount to a very large and shared regulatory sandbox.
- Assessable impact. The project will need to be monitored commensurately with its scale, public visibility, and experimental nature. Participants will need to assess progress toward both defined project goals and broader impact.
- A multistakeholder effort. Considering its public importance and the resources it should marshal, the project will need to be government-initiated. But the architecture and governance should be open to nongovernmental participation on a shared basis.
This proposal could be modeled on several large-scale international scientific collaborations: CERN, the Human Genome Project, or the International Space Station. It would also build on numerous initiatives toward collaborative research and development on AI. Similar global collaboration will be more difficult in a world of increased geopolitical and economic competition, nationalism, nativism, and protectionism among governments that have been key players in these efforts.
Recommendations
Below, we present recommendations for developing international cooperation on AI based on our discussions and work to date.
R1. Commit to considering international cooperation in drafting and implementing national AI policies.
This recommendation could be implemented within a relatively short timeframe and initially would take the form of firm declarations by individual countries. Ultimately this could lead to a joint declaration with clear commitments on the part of the governments involved.
R2. Refine a common approach to responsible AI development.
This type of recommendation requires enhanced cooperation between FCAI governments, which can then provide a good basis for incremental forms of cooperation.
R3. Agree on a common, technology-neutral definition of AI systems.
FCAI governments should work on a common definition of AI that is technology-neutral and broad. This recommendation can be implemented in a relatively short term and requires joint action by FCAI governments. The time to act is short, as the rather broad definition given in the EU AI Act is still undergoing the legislative process in the EU and many other countries are still shaping their AI policy frameworks.
R4. Agree on the contours of a risk-based approach.
Alignment on this key element of AI policy would be an important step towards an interoperable system of responsible AI. It would also facilitate cooperation among FCAI governments, industry, and civil society working on AI standards in international SDOs. General agreement on a risk-based approach could be achieved in the short term; developing the contours of a risk-based classification system would probably take more time and require deeper cooperation among FCAI governments as well as stakeholders.
R5. Establish “redlines” in developing and deploying AI.
This may entail an iterative process. FCAI governments could agree on an initial, limited list of redlines such as certain AI uses for generalized social scoring by governments; and then gradually expand the list over time to include emerging AI uses on which there is substantial agreement on the need to prohibit use.
R6. Strengthen sectoral cooperation, starting with more developed policy domains.
Sectoral cooperation can be organized on relatively short timeframes starting from sectors that have well-developed regulatory systems and present higher risks, such as health care, transport and finance, in which sectoral regulation already exists, and its adaptation to AI could be achieved relatively swiftly.
R7. Create a joint platform for regulatory learning and experiments.
A joint repository could stimulate dialogue on how to design and implement sandboxes and secure sound governance, transparency, and reproducibility of results, and aid their transferability across jurisdictions and categories of users. This recommended action is independent of others and is feasible in the short term. It requires soft cooperation, in the form of a structured exchange of good practices. Over time, the repository should become richer in terms of content, and therefore more useful.
R8. Step up cooperation and exchange of practices on the use of AI in government.
FCAI governments could set up, either as a stand-alone initiative or in the context of a broader framework for cooperation, a structured exchange on government uses of AI. The dialogue may involve AI applications to improve the functioning of public administration such as the administration of public benefits or health care; AI-enabled regulation and regulatory governance practices; or other decision-making and standards and procedures for AI procurement. This recommended action could be implemented in the short term, although collecting all experiences and setting the stage for further cooperation would require more time.
R9. Step up cooperation on accountability.
FCAI governments could profit from enhanced cooperation on accountability, whether through market oversight and enforcement, auditing requirements, or otherwise. This could combine with sectoral cooperation and possibly also with standards development for auditing AI systems.
R10. Assess the impact of AI on international data governance.
There is a need for a common understanding of how data governance rules affect AI R&D in areas such as health research and other scientific research, and whether they inhibit the exploration that is an essential part of both scientific discovery and machine learning. There is also need for a critical look at R&D methods to develop a deeper understanding of appropriate boundaries on use of personal data or other protected information. In turn, there is also a need to expand R&D and understanding in privacy-protecting technologies that can enable exploration and discovery while protecting personal information.
R11. Adopt a stepwise, inclusive approach to international AI standardization.
A stepwise approach to standards development is needed to allow time for technology development and experimentation and to gather the data and use cases to support robust standards. It also would ensure that discussions at the international level happen once technology has reached a certain level of maturity or where a regulatory environment is adopted. To support such an approach, it would be helpful to establish a comprehensive database of AI standards under development at national and international levels.
R12. Develop a coordinated approach to AI standards development that encourages Chinese participation consistent with an industry-led, research-driven approach.
There is currently a risk of disconnect between growing concern among governments and national security officials alarmed by Chinese engagement in the standards process on the one hand, and industry participants’ perceptions of the impact of Chinese participation in SDOs on the other. To encourage constructive involvement and discourage self-serving standards, FCAI participants (and likeminded countries) should encourage Chinese engagement in international standards setting while also agreeing on costs for actions that use SDOs strategically to slow down or stall standards making. This can be accomplished through trade and other measures but will require cooperation among FCAI participants to be effective.
R13. Expand trade rules for AI standards.
The rules governing use of international standards in the WTO TBT Agreement and free trade agreements are limited to goods only, whereas AI standards will apply mainly to services. New trade rules are needed that extend rules on international standards to services. As a starting point, such rules should be developed in the context of bilateral free trade agreements or plurilateral agreements, with the aim to make them multilateral in the WTO. Trade rules are also needed to support data free flow with trust and to reduce barriers and costs to AI infrastructure. Consideration also should be given to linking participation in the development of AI standards in bodies such as ISO/IEC, with broader trade policy goals and compliance with core WTO commitments.
R14. Increase funding for participation in SDOs.
Funding should be earmarked for academics and industry participation in SDOs, as well as for SDO meetings in FCAI countries and more broadly in less developed countries. Broadened participation is important to democratize the standards making process and strengthen the legitimacy and adoption of the resulting standards. Hosting meetings of standards bodies in diverse countries can broaden exposure to standards-setting processes around AI and critical technology.
R15. Develop common criteria and governance arrangements for international large-scale R&D projects.
Joint research and development applying to large-scale global problems such as climate change or disease prevention and treatment can have two valuable effects: It can bring additional resources to the solution of pressing global challenges, and the collaboration can help to find common ground in addressing differences in approaches to AI. FCAI will seek to incubate a concrete roadmap on such R&D for adoption by FCAI participants as well as other governments and international organizations. Using collaboration on R&D as a mechanism to work through matters that affect international cooperation on AI policy means that this recommendation should play out in the near term.
Proposed future topics for FCAI dialogues– Scaling R&D cooperation on AI projects. |